Andrew M. Saxe
2025 United Kingdom Award Finalist — Faculty
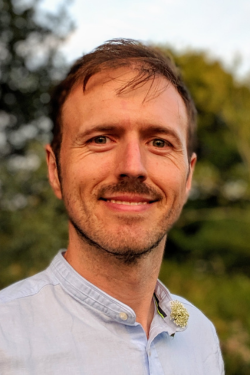
Current Position:
Professorial Research Fellow
Institution:
University College London
Discipline:
Neuroscience
Current Position:
Professorial Research Fellow
Institution:
University College London
Discipline:
Neuroscience
Recognized for: Mathematical analyses illuminating learning mechanisms in artificial and biological systems, advancing AI understanding and insights into memory-related neurological diseases.
Areas of Research Interest and Expertise:
Neuroscience, psychology, machine learning, statistical physics
Previous Positions:
Research Summary:
Neuroscientist Andrew M. Saxe, PhD uses mathematics to understand artificial deep learning, a foundation of modern AI. By elucidating how neural networks learn, Saxe has developed mathematical theories that apply to both artificial neural networks and neurobiological systems. Saxe’s work successfully models complex behaviour such as how children acquire knowledge, how and when neural networks generalise knowledge to new scenarios, and how long-term memories are formed, advancing our knowledge of the brain and how to build artificial intelligence.
“Discovering fundamental mathematical rules that shape how both machines and living creatures learn could have transformative impacts on society, addressing one of science's most profound contemporary challenges. I'm grateful for the recognition of our work in this field.”
Key Publications:
Other Honors:
2023 Blavatnik Awards for Young Scientists in the UK Finalist in Life Sciences
2022 Schmidt Science Polymath Award, Schmidt Futures
2020 CIFAR Azrieli Global Scholarship, CIFAR
2019 Wellcome-Beit Prize, Wellcome Trust
2016 Robert J. Glushko Outstanding Doctoral Dissertations Prize, Cognitive Science Society
In the Media:
Quanta Magazine – The usefulness of a memory guides where the brain saves it