Erik Henning Thiede
2021 Regional Award Finalist — Post-Doc
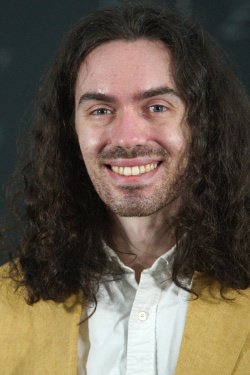
Current Position:
Flatiron Research Fellow
Institution:
Flatiron Institute
Discipline:
Theoretical Chemistry
Current Position:
Flatiron Research Fellow
Institution:
Flatiron Institute
Discipline:
Theoretical Chemistry
Recognized for: Bridging the fields of chemistry and computer science, theoretical chemist Erik Henning Thiede is revolutionizing how scientists simulate the behavior of molecules by using machine learning to make simulations more efficient and accurate. Thiede is designing specific neural networks—artificial decision models that mimic neurons in the brain—that reflect the geometric properties of real molecules. These new tools will make it possible to simulate entire systems of molecules with unprecedented accuracy, giving the clearest view of molecular chemistry ever achieved. Thiede’s work will accelerate the development of new chemistries for chemical synthesis, energy storage, and drug delivery.
Areas of Research Interest and Expertise: Theoretical Chemistry, Computer Science, Machine Learning
Previous Positions:
BS, University of North Carolina at Chapel Hill
PhD, University of Chicago (Advisors: Aaron Dinner and Jonathan Weare)
Postdoctoral Scholar, University of Chicago (Advisor: Risi Kondor)
Research Summary:
By incorporating machine learning techniques—the ability of a computer to learn how to complete a task—into molecular modeling, Erik Henning Thiede, PhD, is building the theoretical framework necessary to simulate molecular chemistry with unprecedented levels of clarity. Simulating and modeling the behavior of atoms and molecules can tell us how a chemical reaction takes place, how energy can be stored in a chemical system, or how drugs are delivered to diseased cells. Thiede has developed a method known as the Dynamical Galerkin Approximation that allows researchers to predict the properties of reactions that happen orders of magnitude more slowly than any single simulation, making it valuable not only to simulating molecules but also fluids and geological processes.
Thiede is now working to make conventional molecular modeling much more efficient and accurate by building machine learning into the modeling process. He is designing novel neural networks that incorporate the specific properties and symmetries of real molecules. By matching the symmetries already present in nature, these neural networks can simulate the behavior of molecules with much less computing power, which leads to more accurate results. The tools Thiede is developing will enable scientists to simulate large systems of molecules, giving scientists detailed insights into how real chemical applications—from chemical manufacturing to energy storage and therapeutic drugs—can be improved.
"Theoretical chemists take the pristine beauty of mathematics and use it to wrangle a messy puddle of atoms into order. When we succeed in bringing this beauty into the world of atoms, we help equip humanity to repair the brokenness of our own world."
Key Publications:
Other Honors:
2017 | MoISSI Software Fellow, Molecular Sciences Software Institute |
2013 | Freud Fellow, University of Chicago |