Jiaoyang Huang
2022 Regional Award Finalist — Post-Doc
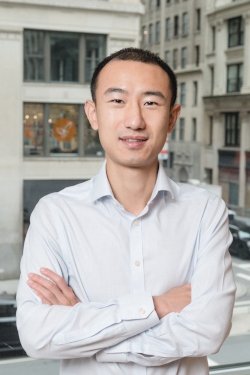
Current Position:
Assistant Professor
Institution:
University of Pennsylvania (previously, New York University)
Discipline:
Applied Mathematics
Current Position:
Assistant Professor
Institution:
University of Pennsylvania (previously, New York University)
Discipline:
Applied Mathematics
Recognized for: Tackling the most fundamental questions that lie at the heart of Random Matrix Theory—a mathematical theory that has widespread use in modern fields of science and engineering.
His work further develops random matrix theory to resolve long-standing questions concerning the spectrum of sparse random graphs, which has profound implications in graph theory and network theory. Huang has also made fundamental breakthroughs in machine learning. He developed a neural tangent hierarchy framework that makes it possible to study the training dynamics of deep neural networks with characteristics that are reflective of real-world, scalable systems.
Areas of Research Interest and Expertise: Random Matrix Theory, Random Graph Theory, Applied Math, Mathematical Physics, Statistical Learning Theory
Previous Positions:
BS, Tsinghua University, China
BS, Massachusetts Institute of Technology
PhD, Harvard University (Advisor: Horng-Tzer Yau)
Member, Institute for Advanced Study
Simons Junior Fellow, Courant Institute, New York University
Research Summary:
Jiaoyang Huang, PhD, has established himself as an exceptional young mathematician working on random graphs and interacting particle systems, which are important topics in Random Matrix Theory (RMT), a theory first developed in the 1950’s to understand the energy spectrum of heavy atoms such as Uranium. His contributions include further development of random matrix theory to the sparse setting, which allows mathematicians to answer fundamental questions concerning the spectral properties of sparse random graphs—a mathematical subject that sits at the intersection of graph theory and probability theory. His recent work characterizes asymptotic behaviors of large families of interacting particle systems and statistical physics models and shows the convergence to random matrix statistics. His remarkable results and influence across the field of mathematics will have impacts well-beyond the field, as RMT has found widespread applications in many modern and seemingly divergent fields of engineering and science. RMT theories describe random fluctuations in systems exhibiting chaotic behaviors, define evolution models for complex systems, and even help illuminate the logistics of bus transportation systems and airline boarding.
Huang has also made fundamental breakthroughs in the fields of data science and machine learning. Using high-dimensional statistics, Huang has developed a framework called the neural tangent hierarchy. It captures the dynamical properties of deep neural networks (computer networks that are modeled after the human brain that predict and perform analytics. His work in this area now makes it possible to study the training dynamics of deep neural networks with practical size.
Develop new tools and techniques in random matrix theory and open the door for more applications to mathematical physics, statistics and machine learning.
Key Publications:
Other Honors:
2020 | Simons Junior Fellow |
2018 | Harvard Graduate Society Term-time Research Fellowship |
2013 | Top 25 of Putnam competition |
2009 | Gold medal in the 50th International Mathematical Olympiad |