Micah Goldblum
2023 Regional Award Finalist — Post-Doc
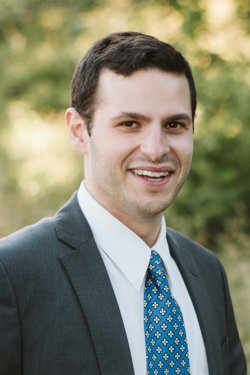
Current Position:
Postdoctoral Researcher
Institution:
New York University
Discipline:
Computer Science
Current Position:
Postdoctoral Researcher
Institution:
New York University
Discipline:
Computer Science
Recognized for: Substantial contributions to various aspects of deep learning—a leading technique of artificial intelligence. His work has not only transformed our understanding of the foundations of deep learning, but also improved its data security. Goldblum also broadened the application of deep learning in data-scarce situations, such as leveraging large volumes of diagnostic data for common diseases to improve diagnoses on rare ones.
Areas of Research Interest and Expertise: Machine Learning; Artificial Intelligence; Security; Computer Vision; Natural Language Processing
Previous Positions:
Research Summary:
AI and machine learning techniques are rapidly shaping our society, but they have also given rise to many questions and concerns. Micah Goldblum, PhD, has made significant contributions in addressing these issues and enhancing our understanding of deep learning—a leading machine learning method. His work not only benefits fellow scientists but also has practical implications for all of us.
One area in which Goldblum has made profound contributions is the foundations of deep learning. He has provided fresh insights into the intrinsic limits of its capability. Recently, he developed a new theory that sheds light on the inadequacy of marginal likelihood—a conventional metric used in selecting model classes for machine learning. To rectify this problem, he introduced a novel variant of the marginal likelihood that effectively aids in deep learning model selection and can be easily computed.
The security vulnerability is a critical concern in deep learning, as even minor alterations to the input or training data by adversaries can exert substantial control over the behavior of trained models. These issues, known as adversarial attacks and data poisoning, have been largely overlooked by many industrial and governmental practitioners. Goldblum's research on adversarial attacks and data poisoning, presented at top machine learning conferences, has highlighted the severity of this problem. Furthermore, he has developed state-of-the-art defenses against such attacks, prompting significant attention from major tech companies and banks to assess the security and stability of products built on deep learning.
Goldblum has also explored the transferability of deep learning models from data-rich scenarios to real-world applications with limited data availability. This research holds immense potential in fields like medical diagnosis, where leveraging data from common diseases can enhance the accuracy of diagnoses for rare conditions.
"I work on fundamental problems in machine learning, such as making neural networks safe and fair or building models that can reason, and at the same time understanding how and why practical systems work using both theory and experiments."
Key Publications:
Other Honors:
2022 Outstanding Paper Award, International Conference on Machine Learning (ICML)
In the Media:
Der Spiegel Magazine – Wie ich die Kontrolle uber mein Gesicht verlor
El Pais – Cómo evitar que los sistemas de reconocimiento facial descifren las fotos de tus redes
The Register – LowKey cool: This web app will tweak your photos to flummox facial-recognition systems, apparently