Ronen Eldan
2022 Israel Award Winner — Faculty
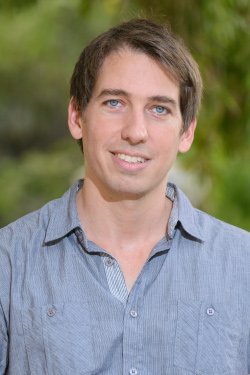
Current Position:
Associate Professor, Department of Mathematics
Institution:
Weizmann Institute of Science
Discipline:
Applied Mathematics
Current Position:
Associate Professor, Department of Mathematics
Institution:
Weizmann Institute of Science
Discipline:
Applied Mathematics
Recognized for: Groundbreaking contributions to high dimensional probability, a mathematical subject that deals with datasets with a very large number of variables. He has not only solved long-standing, open conjectures in this area, but has also developed methods and techniques that have found wide applications in statistics and computer science, most notably in the theory of machine learning.
Areas of Research Interest and Expertise: High Dimensional Probability, Convex and Discrete Geometry, Stochastic Calculus, Machine Learning, Data Analysis
Previous Positions:
BSc, Open University of Israel
BSc, Tel Aviv University
PhD, Tel Aviv University
Postdoctoral Researcher, Weizmann Institute of Science
Postdoctoral Researcher, Microsoft Research Lab – Redmond, USA
Postdoctoral Researcher, University of Washington, USA
Research Summary:
The work of Ronen Eldan, PhD, has led to breakthroughs in solving mathematical conjectures that have profound impacts on the fields of statistics, machine learning, and theoretical computer science. His research spans several fields of mathematics, with a focus on high dimensional probability. This subject area deals with the understanding of random systems in which the datasets have many variables or sources of randomness. It aims to find ways to tame the “curse of dimensionality” which refers to the fact that the number of configutations of a system grows exponentially as the number of variables increases making it difficult to analyze or visualize high-dimensional systems.
One of Eldan’s main research endeavors has been to establish connections between the theory of high dimensional phenomena and stochastic calculus, the theory that explains the motion of diffusing particles. These connections have led to an emerging methodology in the field, referred to as “pathwise analysis”. Eldan first developed a new technique, now coined as “Eldan’s stochastic localization”, which can be used obtain insights regarding the behavior of high dimensional distributions. This technique has proved essential towards solving two central problems in the field of convex geometry that have remained unsolved since the 1980s. These two problems—the hyperplane conjecture by Jean Bourgain and the Kannan-Lovász-Simonovits conjecture—reveal some of the fundamental facts about the geometry of high dimensional convex sets. This progress not only opens the door to solve other questions in high dimensional probability, but also gives important insights in the theoretical foundation of machine learning and data science. By further developing the “pathwise analysis” methodology, Eldan was able to solve two conjectures by prominent mathematician Michel Talagrand, and gain beneficial insights in several fields adjacent to high-dimensional probability such as the theory behind processing of noisy data in statistics, the analysis of Boolean functions—a fundamental object in theoretical computer science —as well as for understanding the behavior of many interacting particles in mathematical physics.
On the applied side, Eldan has utilized the theory of high-dimensional phenomena in two main directions. One of them is to understand the limitations of neural networks in terms of its ability to express functions and fit data, and the role of a network’s depth in those limitations. The other one is to provid the first optimal algorithm for the “convex bandit optimization” problem, a central paradigm for decision-making under uncertainty which combines aspects from optimization and reinforcement learning.
"Science is perhaps the only domain regarding which we can say with high certainty that humanity is actually moving forward."
Key Publications:
Other Honors:
2021 | Invited Speaker at the International Congress of Mathematicians 2022 |
2018 | Erdös Prize, Israel Mathematical Union |
2013 | Haim Nessyahu Prize, Israel Mathematical Union |
In the Media:
Weizmann Wonder Wander – Taming the Curse of High Dimensionality