An idea that, in retrospect, led to the solution of several problems in high-dimensional geometry, including a riddle that has preoccupied mathematicians for many years, popped into the mind of Prof. Ronen Eldan, 41, on his way to buy falafel in Tel Aviv. "I came back from university on my bike," he recalls, "and on the corner of King George and Zamenhof streets there was a moment when I said to myself, ‘Hey, maybe I should try to check if this method leads to something’”. It was not a eureka moment, he explains, but more of a preliminary idea in a direction that might yield something.
"As a mathematician, a big part of the work and research is to try to find insights in unrelated theory and weave them into your theory," he says. The idea that Prof. Eldan incorporated into his field of study—high dimensional geometry and probability—is based on the mathematical principles that explain Brownian motion (diffusion of particles). It was only when he began to further develop the idea later that evening and in the days that followed, that Prof. Eldan realized that this idea seems to lead to a breakthrough in the field he was researching, regarding a question that has important implications in the field of data science.
Nine years later, it was this breakthrough that contributed to the selection of Prof. Eldan as the Physical Sciences & Engineering Laureate of the 2022 Blavatnik Awards for Young Scientists in Israel. Prof. Eldan will receive US$100,000 in unrestricted funds, join the prestigious list of Blavatnik Awards honorees in Israel and around the world, and participate in meetings and symposia with other winners of the Blavatnik Awards for Young Scientists, which operates in the United States, the United Kingdom, and Israel. He is the first pure mathematician to win the Israel Award, which was launched in 2017 in a collaboration between the Blavatnik Family Foundation, the Israel Academy of Sciences and Humanities, and the New York Academy of Sciences.
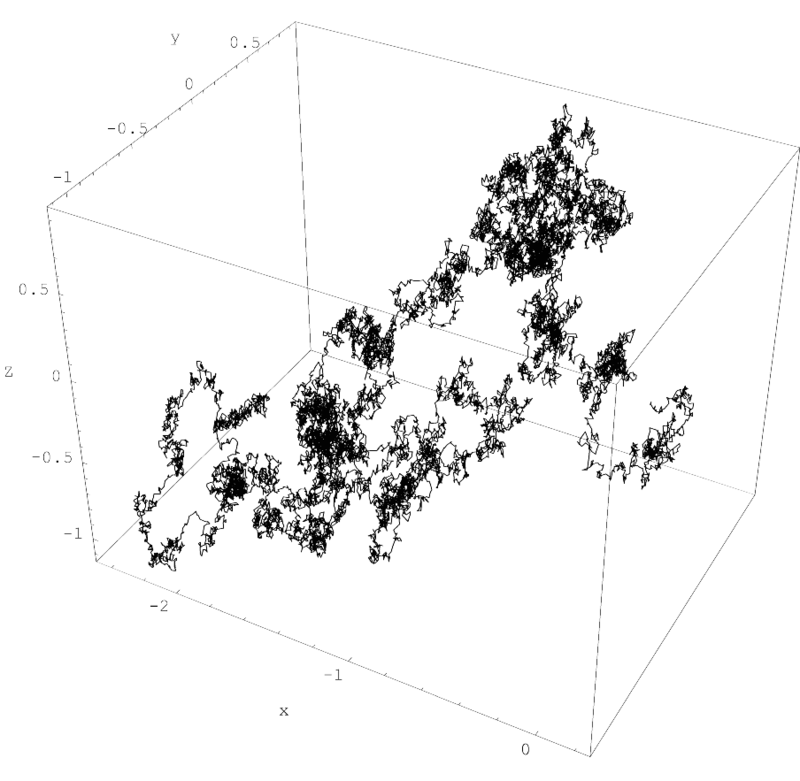
A single realization of three-dimensional Brownian motion. Source: https://en.wikipedia.org/wiki/Brownian_motion#/media/File:Wiener_process_3d.png
The field of theoretical mathematics that Prof. Eldan is researching—phenomena in high-dimensional systems—is almost impossible to explain in a short text, he says, but he is willing to try nonetheless. Prof. Eldan says that, in short, his research deals with the geometry and probability of objects in high-dimensional spaces.
We are all familiar with a circle (two-dimensional body) or a sphere (three-dimensional body), but what about objects in higher dimensional spaces? "Most of the geometric objects we know have multidimensional versions," explains Prof. Eldan, "and they can be very relevant to our world. Imagine, for example, all the blood test results of insureds in a particular health care plan, each of which consists of 50 different results. They can be treated as a dataset in a space where there are 50 dimensions—the number that indicates the number of 'coordinates' required to place a point in space."
"Within this group of objects," says Prof. Eldan, "one can speak of a subset consisting of the results of tests that indicate a particular medical condition, such as diabetes. Understanding the geometry of such a subset has direct implications for our ability to identify diabetes from all the tested insureds in the future, because this understanding gives insights into the question of whether I can study this subset using machine learning." He says. "Understanding its geometry allows me to give the algorithm some features that actually tell what kind of objects to look for, and in what class.", says Prof. Eldan, but adds that this understanding is not only practical, but has pure mathematical importance.
"Basically, ‘big data’ is statistics that operate in high-dimensional spaces," explains Prof. Eldan, "but instead of coordinates, we enter data. In fact,” he adds, "almost everything we do in data science and machine learning—developing algorithms that allow a computer to learn from examples—can be phrased as a mathematical problem regarding high-dimensional objects.
Using Prof. Eldan's methodology, it is possible to apply the theory of Brownian motion to the analysis of objects of high dimensions, thus enabling a new point of view that leads to insights into their behavior. "My methodology utilizes a seemingly-unrelated theory, which concerns the diffusion of particles," explains Prof. Eldan. "We use the theory of diffusion to help describe objects of high dimensions." Prof. Eldan says that through application of the method he developed, he can understand “several basic aspects related to the question of what a set that lies in a high-dimensional space looks like."
Despite the abstract thinking required to understand this scientific achievement, some of the discoveries themselves have very practical implications. For example, Prof. Eldan’s new methodology has led to the solution of one of the major problems in the field of high-dimensional geometry: Bourgain’s slicing problem (named after the Belgian mathematician Jean Bourgain). In addition, the method has direct implications for machine learning: "It helps to show that machine learning algorithms that have already been designed can solve a larger set of problems and can also be used to develop new algorithms," he explains.
Prof. Eldan is currently on sabbatical at Princeton University with his family, and says he received the call informing him that he had won the Blavatnik Award early in the morning. "It was six-o’-clock in the morning, and the main thing that went through my mind was that it would wake my daughter," he says with a smile, and adds he was very excited to hear that he had received the prestigious award.
"I didn't really want to be a mathematician from childhood," he recalls. "I really liked physics and engineering, and as a kid I liked building things." During his military service he began taking mathematics and physics courses at the Open University and eventually completed a degree in mathematics. "I finally focused on mathematics, in part because there were no physics labs at the Open University,” he said. He went on to pursue a master's degree and a doctorate at Tel Aviv University on "Distribution of Mass in Convex Bodies," and then a postdoctoral fellowship at Microsoft's research lab near Seattle. In 2015, he returned to Israel, to serve as a faculty member at the Weizmann Institute of Science.
As a pure mathematician, Prof. Eldan is doing his research without a laboratory or complicated experimental software. "Most of the time it's me with myself, and I just think. It happens while I'm on the train or waiting in line. I work with students, which is perhaps the most fulfilling part of academic life, and I'm sure I would not have gotten anywhere without the mentors I had, but in the end, most ideas arise when you’re sitting by yourself and thinking." When asked if he has ways to force those moments to happen, he jokes, "If I was stuck in a room with exposed concrete walls, no windows, and most importantly no internet, it would be the most effective way for me to make progress in math."